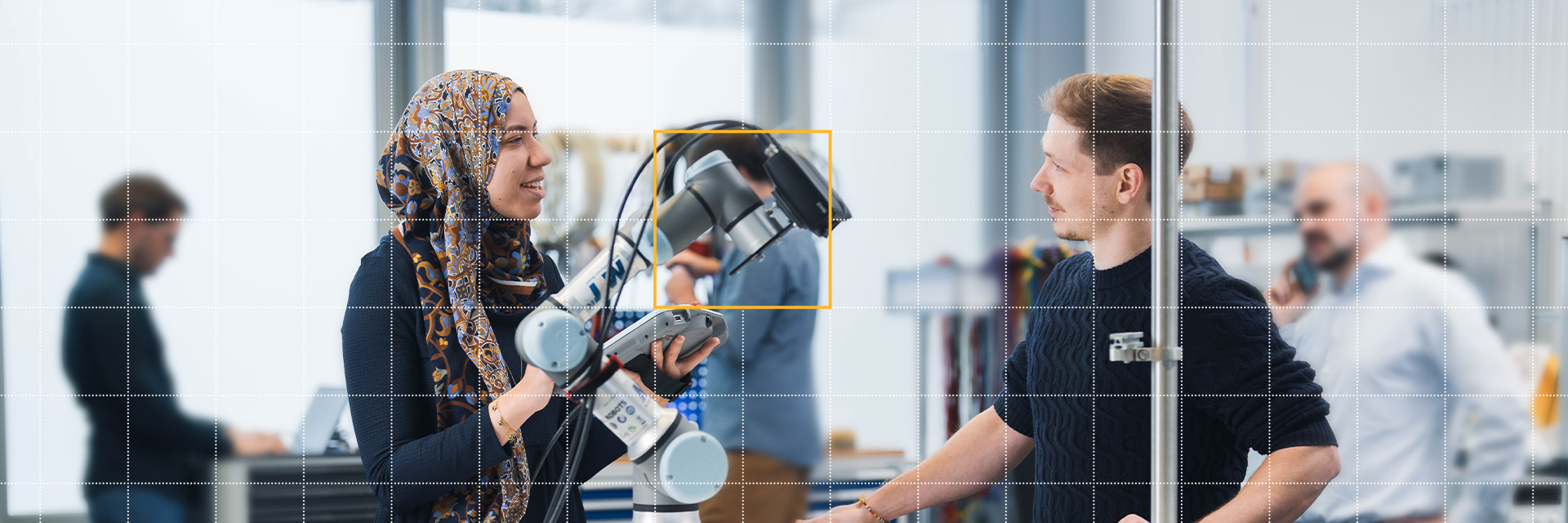
An eye on everything with deep learning
MERLIC | Automotive | Electric Components | Deep LearningBosch Car Multimédia in Portugal specializes in the development and manufacture of electronic components for vehicle safety and dynamics, driver assistance, automated driving and car multimedia. The company uses the machine vision software MERLIC from MVTec Software GmbH to improve the quality inspection of electronic components with its deep learning methods.
High demands on the quality inspection of electrical connections

Bosch Car Multimédia, as a supplier of electronic components for various customers in the automotive industry, depends on a high quality standard of its manufactured automotive sensors. To ensure this standard, defects must be detected quickly and reliably. In this specific case, the task is to check metal springs for defects. These springs form the electronic connection between the main circuit board and a copper bushing on the cover of a sensor. Since this is a manual machining process, different defects can occur on the metal spring during production.
Optimizing quality inspection with machine vision
Bosch already relied on an automated inspection process before, but wanted to further optimize it. The goal was to improve the overall quality of the inspection, make the new solution more cost-effective, and reduce maintenance work for the application. Bosch chose the MERLIC machine vision software from MVTec Software GmbH in Munich. MVTec MERLIC is an easy-to-use machine vision software that makes it possible to solve the most common machine vision applications even without programming skills.
Deep learning helps to detect defects

In Bosch’s application, machine vision looks like this: A five-megapixel camera captures an image from above for each component. Global Context Anomaly Detection is used to inspect the captured images with the metal springs. The deep learning technology has two neural networks. The "local" network checks for small-scale defects such as scratches, cracks or dirt. The "global" network goes one step further and checks for logical defects such as bent or missing brackets. From the interference of the two networks, Global Context Anomaly Detection determines an anomaly score. This value is then compared with the anomaly threshold value defined in advance. If the Anomaly Score is above this threshold, the component is defective and must be rejected. After the inspection, each image can be reviewed in MERLIC’s frontend. Especially helpful: A heat map can be used to transparently trace which parts of the image triggered the anomaly detection.
Until now, the inspection process has been carried out using rule-based machine vision methods. However, one of the disadvantages of this was that all possible types of defects had to be extracted individually using "bad images". In contrast, training the deep learning methods of MERLIC, only requires "good images" of intact parts. Since these are very easy to acquire, it saves time and money.
Integration of machine vision software into customer-specific machine control system

The question of how to integrate machine vision software into an existing production process was particularly exciting for Bosch, since the production process and the integrated quality inspection were not to be changed. Thus, the main focus was on the integration of the machine vision software into the machine control system. The software had to be connected directly to the machines, since the plant does not have a programmable logic controller (PLC). The MQTT protocol integrated in MERLIC provides the necessary machine-to-machine communication. This allowed the machine vision software to be easily integrated into the process via standard IoT communication protocols. The development of the machine vision system's calibration program could be accelerated via the easy-to-use software.
More machine vision projects planned
"We successfully completed the proof-of-concept at the end of 2022. In the process, all of our goals were achieved in terms of detection rates, system maintenance requirements, and costs. Therefore, the commissioning of a new production line will take place in mid-2023. The rollout to other existing lines is then planned afterwards," explains João Paulo Silva, a testing expert from the Center of Competence Optics and Mechanics department at Bosch Automotive Electronics in Portugal. Based on the potential, Bosch plans to automate more Automotive Electronics plants in the future using deep learning.